Like most advertisers, publishers, and AdTech firms, the industry [as a whole] welcomed the news. The stay in deprecation has allotted additional time for our industry to come up with one or several comprehensive solutions to prepare for the impending change.
In their recent statement, Google announced that the company is going to follow all recommendations posed by the European Commission and help publishers to prepare and test various solutions. Skeptics may expect, however, that antitrust requirements by European governments will not suffice to make sure there will be no preference made for Google’s own solutions in the development or implementation of Privacy Sandbox methods.
Despite its shortcomings, 3rd Party Cookies was an acceptable solution for storing, transmitting, using, and monetizing targeting data as well as measuring users’ exposure to ads for frequency capping and attribution purposes. Let’s take a closer look at the AdTech Industry’s current attempts to counter the demise of 3rd Party Cookies.
Alternative identity solutions
With 3rd Party Cookies being rendered absolute, brands, agencies, and tech intermediaries may opt for substitute advertising identifiers. However, let’s agree that it can’t be 3rd Party Cookies by another name. Deterministic non-consented identifiers will eventually raise the same privacy concerns, so they can’t be a long-term cross-industry solution.
So far, identity plans fall into two broad categories, non-cookie based probabilistic non-consented IDs and login-based deterministic consented solutions. The first ones use the users’ IP addresses, the user agent string, and geo locations to establish anonymous identities, whereas the latter ones rely on deterministic and persistent IDs, i.e. users self-identify themselves by logging in.
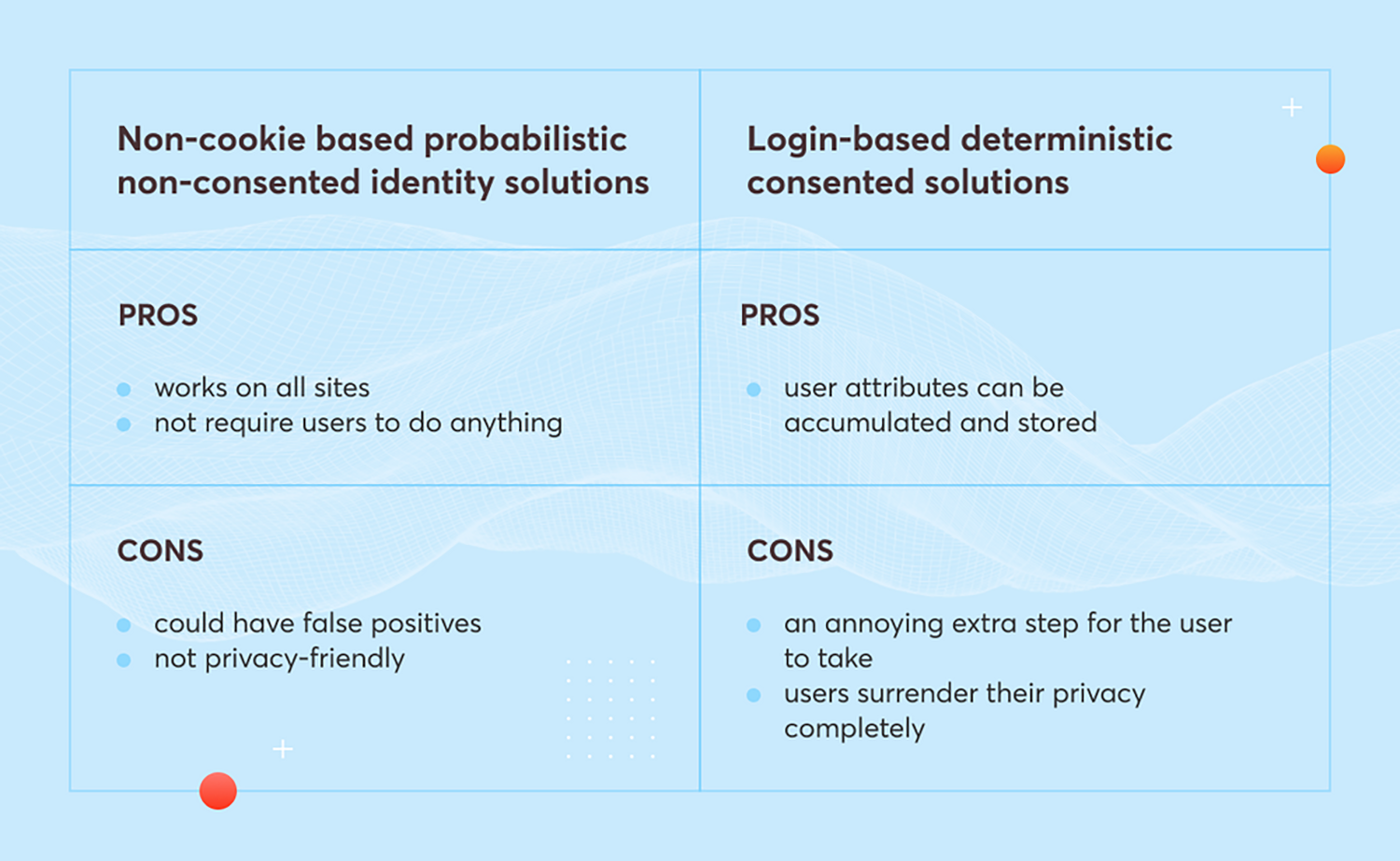
Alternative targeting solutions outside of identity
What if none of the above-mentioned identity solutions succeed? The login-based deterministic solutions might fail because many users will refuse to identify themselves in order to check the local weather or consume daily news. The probabilistic solutions might fail because they are not truly privacy compliant and are rather inaccurate.
MGID and several other companies are enhancing contextual solutions as an alternative to identity-based targeting. While contextual targeting is not new, machine learning-based solutions such as MGID Contextual Intelligence (CI) make contextual targeting far more effective.
Contextual intelligence is 100% privacy-friendly because ads are targeted to what’s on the page and not any user data. The advertised content is relevant to the user intent at the moment. Let’s consider the example of b2b ads: targeting is more effective while the user is consuming business news at work. The user will be more likely to ignore the same ad if they see it when making dinner reservations.
The weak point of these targeting solutions, however, is that there is less contextual data than there is behavioral and profile data. A user can see one page with a particular topic at a time (contextual), but the user’s identifier could be connected to thousands of pages that they visited in the past (behavioral). Thus, the user could be targeted to one contextual but thousands of behavioral categories.
Contextual Intelligence by MGID solves this problem by relating contexts to behaviors. For example, expensive watch brands are not contextually relevant to 1st class travel. The behavior of buying an expensive watch, and the behavior of shopping for a 1st class ticket is somewhat related.
Ways to improve the revenue that do not relate to targeting
The reason why many Publishers are concerned about the upcoming deprecation of 3rd Party Cookies: Revenue. What if there were ways of improving revenue that did not have to do with identity or targeting? Pursuing such improvements would also be a good way to prepare for the Cookiepokalypse.
One possible answer is better optimization tools based on Machine Learning solutions. Being able to identify the user profile or user intent is only half the battle. The other, much more important struggle is to determine what to do with it.
For example, if you are a soft drink company, you may opt to target pages that talk about soft drinks or choose to advertise to user IDs that expressed an interest in soft drinks or both. But the more restrictions you put on targeting, the less scalability you have.
More often than not, you will be dealing with a degree of relevance and various contexts. In Machine Learning specialists’ lingo, “context” is not just a page category, but it could be dozens of other features such as day of the week, time of day, device, browser, page in a session, exposure to similar ads in the past, etc.
In the not-so-distant past, advertisers relied on an army of campaign managers that manually selected targets, frequency caps, and bids for each slice of context. This manual-based approach could deliver optimal results for advertisers but it cannot be easily scaled. As your marketing reach grows progressively, these one-to-one adjustments become a nearly impossible task to do. This is why MGID enhances its optimization capabilities by taking advantage of the latest advances in modeling and machine learning.
Final thought
As Google Chrome accounts for nearly two-thirds of the global browser usage, Google’s decision to make 3rd Party Cookies obsolete remains to be the ultimate tipping point in the demise of this technology. Uncharted waters of the cookieless era can be closer or farther away on the timeline, but all major industry players still have to decide how they are going to enter them.